Research
I primarily focus on two topics, which are the cosmic reionization
and modeling baryonic feedback processes in large-scale observations.
I also develop state-of-art data analysis tools using the latest machine learning methods.
Below I give a highlight of my research interests. Here I list the content of this page:
Cosmic reionization
About 50 million years after the birth of our universe, the first luminous sources formed. I seek signatures of these sources and study how they reionized gas in the intergalactic medium (IGM) of our universe. Above I show an example of the reionization in a simulation volume of with a length of 714 Mpc (see Giri & Mellema 2021 for details). The ionization state of the IGM is shown in the left panel. One can see the ionized regions (black) growing, merging and finally filling the entire universe. One interesting probe of this process is the 21-cm signal, which is produced by neutral hydrogen due to the spin-flip transition of its ground state. The right panel shows the corresponding 21-cm signal. The ionized regions are seen as absence of signal (dark-blue) in the map.
Modelling method
Numerical simulations
We simulate cosmic reionization by post-processing N-body simulation outputs with radiative transfer simulation code. One such code is called C
2Ray (Mellema et al. 2006) that solves the transport of photons from luminous sources in three dimensions. With this method, we can accurately simulate very large simulation volumes (∼1 Gpc) that is very difficult to achieve with hydrodynamical simulation methods (e.g.
Giri et al. 2019b;
Giri & Mellema 2021). The figure below shows slices from such a large-scale reionization simulation.
Analytical simulations
In order to interpret 21-cm signal observations, we need a fast modelling method. In
Schneider, Giri & Mirocha (2021), we introduced a halo-model based approach to model the 21-cm signal. In the figure below, we compare the power spectra derived from our halo-model based approach assuming exponential and extended Press-Schechter halo accretion (solid and dash-dotted lines) with that from 21cmFAST code (colored bands).
The 21-cm signal
The 21-cm signal produced by the neutral hydrogen gas present in the intergalactic medium (IGM) during reionization contains a
treasure-trove of information about the reionization process. Within the Low-frequency Array (LOFAR) Epoch of Reionization
Science team, we are attempting to measure the strength of spatial fluctuations in the signal (or the power spectrum). We have
provided the tighest upper limit at a redshift of 9 (Mertens et al. 2020)
and constrained the properties of the IGM using this observation (Ghara, Giri et al. 2020).
In the right panel above, we show the LOFAR upper limits (red points) along with many reionization models (coloured lines).
We see that there are some model that violate the observed upper limits. In the left panel, we show a slice from an excluded model model
(blue dashed power spectrum given in the right panel).
Going beyond the power spectrum
The 21-cm signal from the epoch of reionization is highly non-Gaussian. Therefore the power spectrum cannot completely characterise
this signal. We have studied various metrics that can probe the non-Gaussian information
(see my PhD thesis
for more discussion). Here I show few such summary statistics that can be estimated from the SKA-like image data.
Bubble size distributions
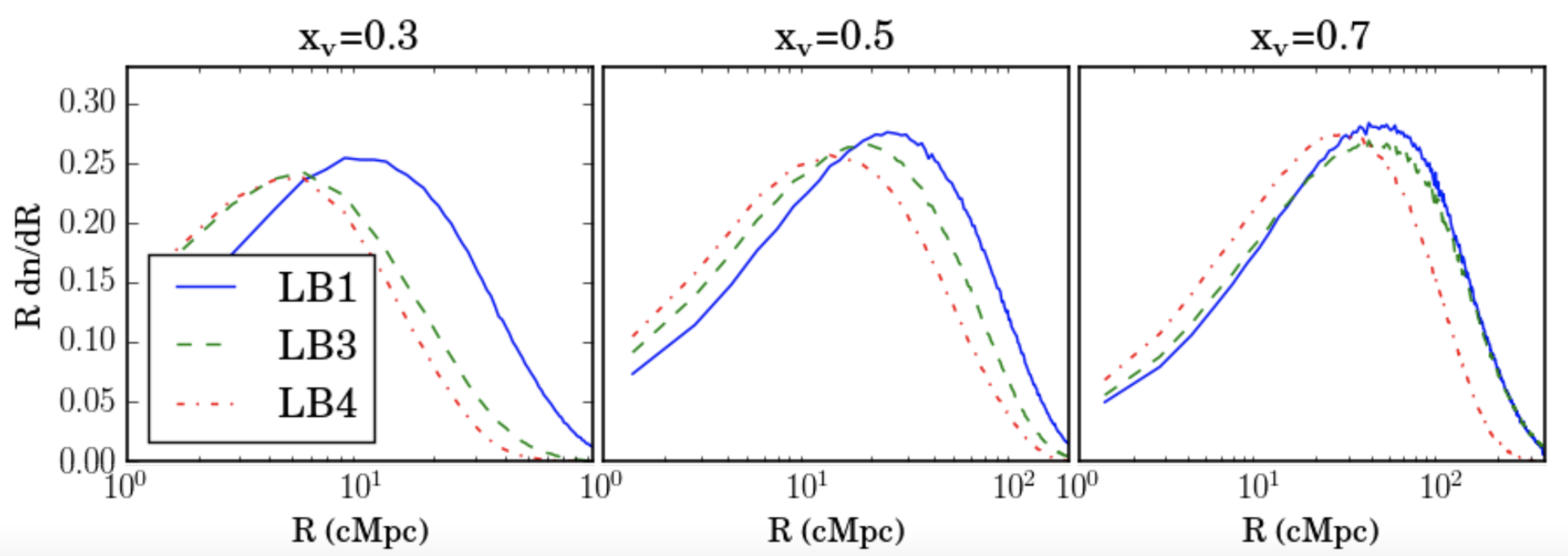
The bubble size distribution (BSD) measures the probability of sizes of the ionized regions (bubbles) during reionization. The BSD shows the growth of these bubbles
at different epochs of reionization. The above figure in taken from
Giri et al. (2018a),
where the BSDs are shown for different models of reionization.
Betti numbers
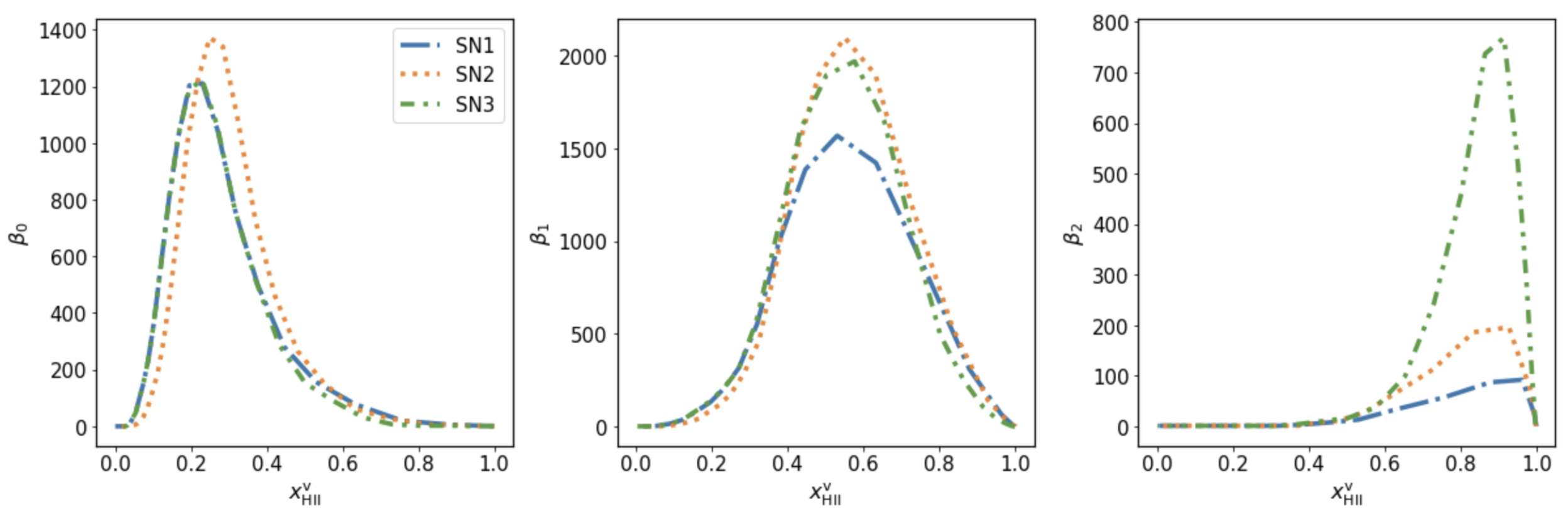
The Betti numbers quantify the morphology of different structures. In
Giri & Mellema (2021),
we used the Betti numbers to study the morphology of ionized regions identified in 21-cm image data. The zeroth, first and second Betti numbers give the number
of isolated structures, tunnels and enclosed voids respectively. The above figure taken from
Giri & Mellema (2021)
shows the evolution of the three Betti numbers as reionization progresses in three different models.
Position dependent power spectrum
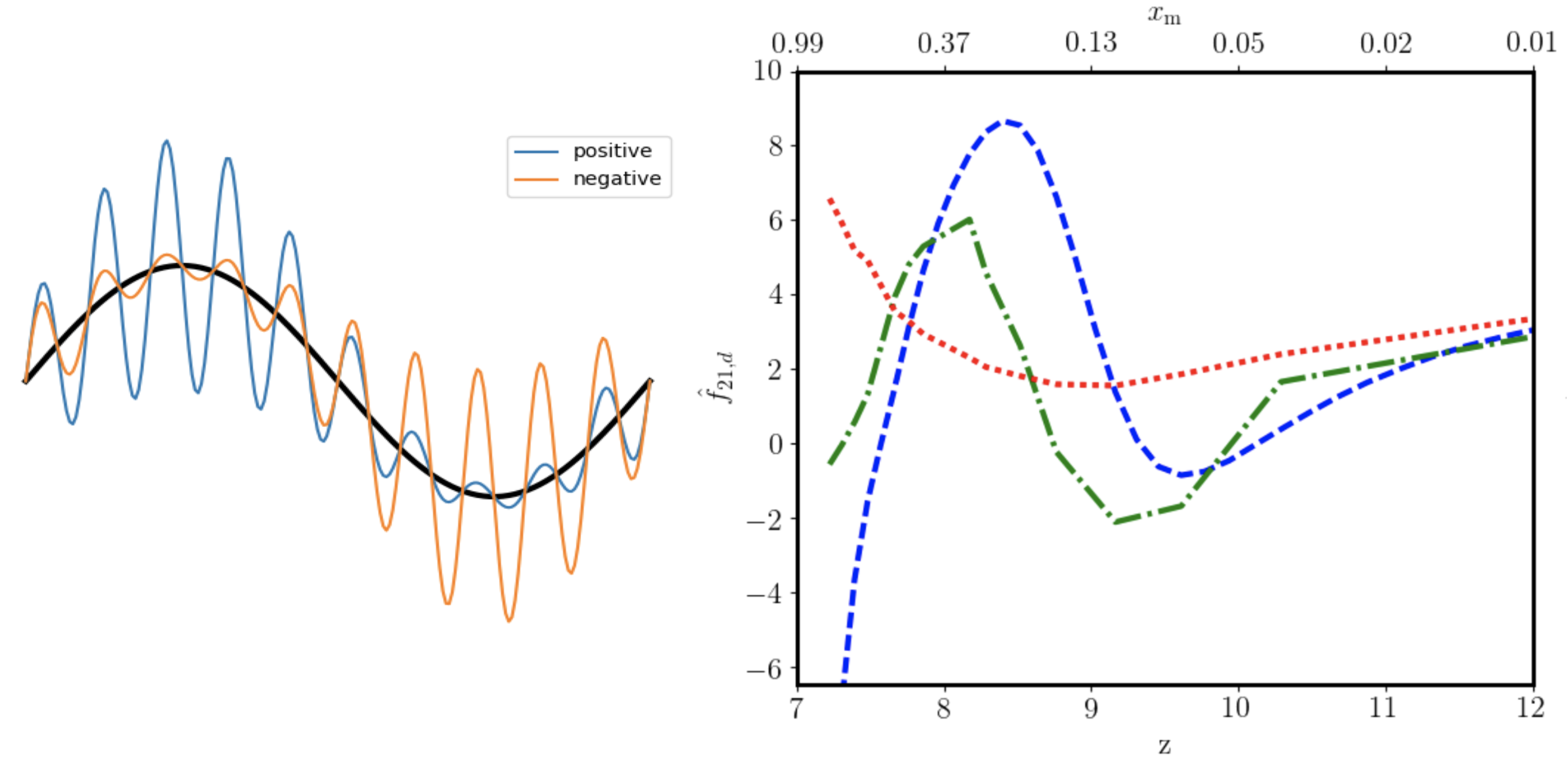
The Position dependent power spectrum (PdPS) measures the response of small-scale fluctuations to the large-scale fluctuations, which is pictorially
shown in the left panel of the above figure. PdPS is mathematically equivalent to the integrated squeezed-limit bispectrum. The right panel of the above
figure is taken from
Giri et al. (2019a) for various reionization models.
Bispectrum
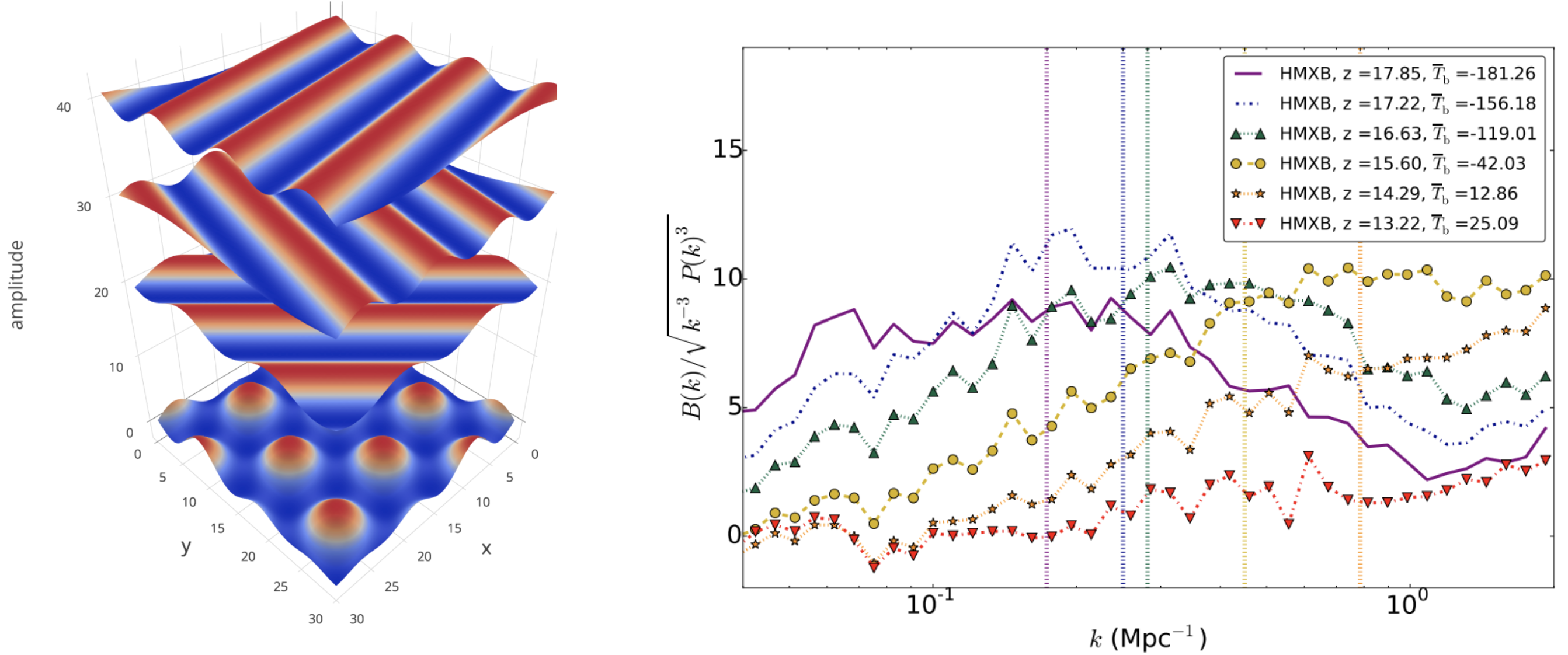
The bispectrum is a higher-order summary statistics. It is the Fourier counterpart of the three point correlation function. The above figure is taken from
Watkinson et al. (2019). The left panel gives a visual representation of the structures probed
by the bispectrum and the right panel gives the equilateral bispectrum calulated from the simulations of the time when first sources formed and heated the
gas in the IGM.
Baryonic feedback process
I also work on understanding the small-scale baryonic feedback processes that affects the observations of large-scale structures, such as the weak-lensing
observations.
Modelling method
The dark matter around dark matter haloes (where the visible objects, such as galaxies and clusters, reside) follow the
NFW profile (Navarro, Frenk & White 1996).
Due to the astrophysical processes inside haloes, the baryons are redistributed and thus do not follow the NFW profile (e.g. van Daalen et al. 2011).
We model the impact of baryonic feedback processes on large-scale observations by parameterising the density of baryons around haloes (see figure below). These profiles can be constrained by galaxy observations
(e.g. Schneider et al. 2019; Debackere et al. 2020; Giri & Schneider 2021).
In N-body simulations
We displace the particles in dark matter (N-body) simulations outputs such that the matter profiles match the baryonic profile.
In this process, we introduce new parameters that describe the baryonic profile. For details, see
Schneider & Teyssier (2015).
This method is computationally inexpensive compared to hydrodynamical simulations. We can derive the large-scale observables,
such as the power spectrum and bispectrum, from the dispalced N-body simulations. In the figure below (taken from
Giri & Schneider 2021),
we show the ratio of power spectra from dark-matter-baryon and dark-matter-only simulations (power spectrum suppressions) at two redshifts. Here we fit the power spectrum suppressions from
hydrodynamical simulations with our fast method.
Baryonic correction emulators
Even though the modelling of baryonic feedback processes by displacing N-body simulations is computationally cheap, it is not fast enough to be used in a statistical inference framework to analyse observations. Therefore emulators of the observables using machine learning methods are helpful in achieving this task (Arico et al. 2021; Giri & Schneider 2021). We build an emulator for modelling the suppression in power spectrum due to baryonic feedback processes, which is publicly available named BCemu. In the figure below (taken from Schneider et al. 2022), we show the constraints on cosmological parameters (S8 and Ωm) using the KiDS-1000 release of weak lensing data. We study the impact of baryonic feedback processes on these constraints by including X-ray and kinematic Sunyaev-Zeldovich observations of galaxy clusters with the weak lensing data.
Machine learning tools
I frequently implement advanced data analysis methods, such as machine learning, in my research work. I am also involved in developing new
machine learning architecture conducive for Big data produced in the field of astronomy.
Analysing high-redshift galaxy spectra
Recently, the JWST started observing the high-redshift galaxies. It will provide us with huge amount of galaxy spectra,
and tranditional analysis methods will be time consuming. Therefore, I developed a machine learning-based framework to
identify Lyman continuum leaking galaxies from large data sets of spectra (Giri et al. 2020).
Building suggorate models
Machine learning-based surrogate models are a very useful way on speeding up any computationally expensive modelling method.
We developed several surrogate models or emulators. Here I list these emulators:
- 21-cm signal power spectrum (Ghara, Giri et al. 2020)
- Impact of baryonic feedback processes on the matter power spectrum (Giri & Schneider 2021)
- Non-standard dark matter models
- Cold+warm dark matter (Parimbelli et al. 2021)
- Decaying dark matter (Bucko, Giri, Schneider 2023)
Structure identification
The upcoming Square Kilometre Array (SKA) will revolutionize the field by producing images of distribution of
neutral hydrogen during reionization using the 21-cm signal. In the left panel of the above image, I show an expected
image from the SKA. An interesting information contained in such images is the ionized regions, where most of the
luminous sources reside. Identifying these regions will not only help us understand the evolution of the reionization
process (Giri et al. 2018a,
2019b; Giri & Mellema 2021)
but also strategise complementary galaxy observations, such as Euclid, James Webb Space Telescope (JWST),
Nancy Grace Roman Space Telescope and Extremely Large Telescope (ELT)
(Zackrisson et al. 2020). We have developed techniques
to identify meaningful features in noisy data (Giri et al. 2018b;
Bianco et al. 2021). In the right panel of the above image,
I show the true ionized regions (red) and the regions identified by one of our methods (green boundaries). These methods along
with the tools to create mock SKA images are packaged into an open-source framework, Tools21cm.
Foreground mitigation for 21-cm signal
One of the most difficult contaminants of the 21-cm signal observations from the epoch of reionization
is the foregrounds that will be orders of magnitude higher in strength. We are developing a machine learning-based
foreground mitigation framework. Currently, we developed SegU-Net v2.0 (Bianco, Giri, et al 2023) that will be able
to identify structures in 21-cm signal image data contaminated with foreground.